Case Study: Kaleidoscope Data uses AI to improve data accuracy by as much as 83%
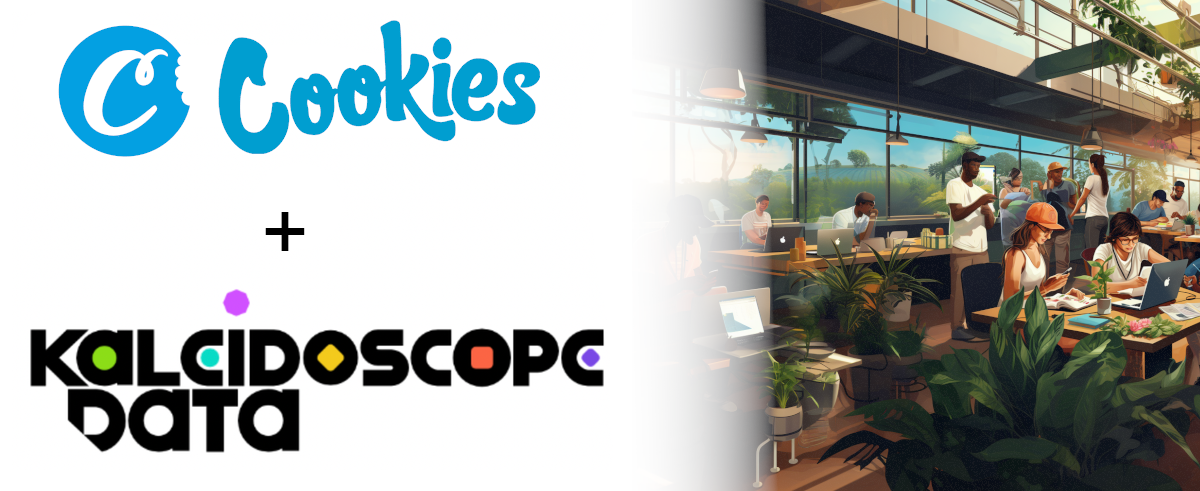
CLIENT
Cookies, the most globally recognized cannabis company, has over 65 retail locations in over 20 markets across 6 countries.
CHALLENGE
Many of Cookies’ retail stores use different point-of-sale (POS) systems. Stores employ inconsistent product nomenclature and data entry formats, resulting in poor data quality across the board — as low as 16% accuracy from the original POS data source.
Cookies needed a partner to vastly improve the accuracy and standardization of data flowing from retail stores to their central data warehouse. Furthermore, the ideal data solution needed to achieve near perfect accuracy with minimal impact on the stores’ existing processes.
SOLUTION PT. 1: ELT PIPELINE & DATA WAREHOUSE
As a first measure, Kaleidoscope Data successfully built and maintained an extract, load, and transform (ELT) pipeline and data warehouse for Cookies in late 2018. The ELT pipeline’s role was to use complex rules and logic to standardize data from retail stores’ POS systems, and then shepherd that data to a central cloud database.
Kaleidoscope’s ELT pipeline made huge improvements in the completeness and accuracy of sales data. For example, the “product name” field increased from 16% to 74% in accuracy, and was populated more often, increasing its completion rate from 58% to 94%.
Despite making huge improvements, there were limitations to just how perfect the data could ever be. To further refine the results would require additional employee hours to do time consuming, ongoing manual review by error-prone humans, or cost-prohibitive, overly complex machine learning solutions.
SOLUTION PT. 2: AI-POWERED DATA CLEANSING
Kaleidoscope revisited this issue in 2023. With access to new Large Language Model (LLM) technology by OpenAI, Kaleidoscope believed it could clean Cookies’ data more efficiently and effectively than before.
Kaleidoscope tested this hypothesis by randomly selecting a sample of data to clean, and were pleasantly surprised that accuracy was near perfect. It quickly became clear that using AI to solve this problem was the best choice since it was very cheap, fast, and accurate, with performance nearly identical to, if not better than that of a human.
Next, Kaleidoscope designed a framework that can accept dirty data as input, apply a precisely defined set of cleaning guidelines, and then generate cleaned data as output. This system has been integrated into the data transformation pipeline and finally into the Cookies’data warehouse environment.
After cleaning all of the existing product data in the system, Kaleidoscope implemented a series of recurring daily tasks which find new entries, clean them, and repopulate the data into the data warehouse. The end solution is a clean database of historic data coupled with an active set of AI-powered filters that ensure all new incoming data is both complete and valid.
Usually, handling input/output is a simple task for software. However, AI models can exhibit apparent randomness in their outputs due to their sensitivity to small changes in the input or parameters, especially in complex models like neural networks. This phenomenon, known as “chaotic behavior,” can make the model’s outputs seem random even though they’re deterministic.
To protect against chaotic behavior and maintain the highest levels of data integrity, Kaleidoscope built additional safeguards in the AI framework and data transformation pipeline.
RESULTS
Kaleidoscope’s AI-driven efforts have yielded vitally important “last mile” improvements to Cookies’ data quality in terms of both completeness and accuracy.
Cookies now can expect all new incoming sales data to be between 94-99% complete, and 97- 99% accurate. This is in stark contrast to the original POS data, which may have been as low as 58% complete and only 16% accurate.
The ability to append data is a major contributing factor to the new process’ success. Whenever a new data entry from a POS contains an empty “product” field, the AI-driven process is able to extract and append a correct, standardized product name from another field 77% of the time. For empty “brand” fields, the AI-driven process can extract and append the correct brand name 91% of the time.
FINAL ANALYSIS
Dirty data creates all types of serious business implications, affecting a company’s decision-making processes, customer relationships, operational efficiency, and even its bottom line.
Kaleidoscope’s strategic implementation of OpenAI’s language model has resulted in a vast improvement in data quality. Cookies can now leverage this sales data in activities such as competitive analysis, demand planning, financial forecasting, and marketing campaigns.
This AI-driven approach to data quality has proven to be a successful and cost-effective solution, and serves as a testament to the potential of AI in driving business growth and efficiency across the retail and cannabis industries.
Need help with something data-related?
Get in touch with us at sales@kaleidoscopedata.com and let’s discuss how we can help transform your business.